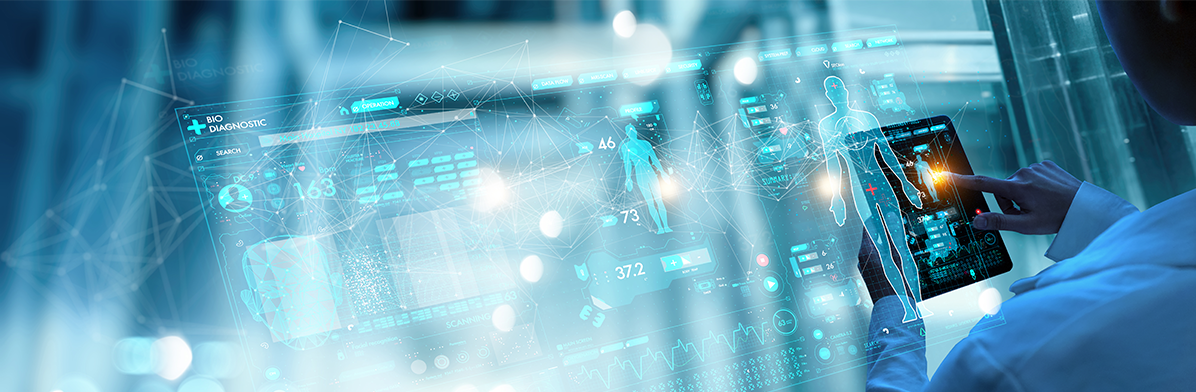
Research meets data protection: Analysing synthetic health data using artificial intelligence
BlogTesaser_Stand
The Health Data Lab (HDL; German: Forschungsdatenzentrum Gesundheit) at the BfArM aims at converting highly sensitive health data into new, "synthetic" datasets for anonymisation purposes. The use of this data is supposed to take place in virtual environments - protected areas where researchers can then examine the data with the help of artificial intelligence.
Big data, machine learning and the use of artificial intelligence (AI) are playing an increasingly important role in healthcare: How can we find the best possible treatment for each patient? How can we recognise the risk factors for severe courses of diseases at an early stage?
In order to answer such questions, medical research needs one thing above all else – a huge amount of data. In the future, such data will be made available to researchers by the Health Data Lab at the BfArM. This involves billing data that is transmitted to the HDL by the German National Association of Statutory Health Insurance Funds in pseudonymised form and contains, e.g., information on diagnoses, treatments and costs. What makes this special is that the data is collected in everyday care settings and is often easier to transfer to the general population than the results from standardised study conditions. Thus, such data provides a basis for scientific publications that contribute to the improvement of medical care.
Protection of sensitive health data
Billing data thus represent a valuable research asset, while at the same time being sensitive personal data to be especially protected. The HDL has to guarantee security of the data in accordance with the state of the art. In order to ensure this, the HDL works together closely with the Federal Office for Information Security (BSI) and the Federal Commissioner for Data Protection and Freedom of Information (BfDI).
As a rule, the data transmitted by the health insurance funds is already pseudonymised. Without additional information, they can therefore no longer be assigned to a specific person. Unique identifiers such as names, addresses or telephone numbers are of course not transmitted. The project "Artificial Intelligence at the Health Data Lab - Investigation of anonymisation methods and AI-readiness", is now investigating a further step towards data protection: the question is whether this so-called synthetic data can be used as a high-quality alternative to anonymised original data.
Research with synthetic data in virtual environments
What is "synthetic data"? Synthetic data is generated artificially, e.g., by training machine learning algorithms on the information and structure of original datasets. The resulting data retain the statistical properties of the original data. However, they no longer contain any real information about the patients, making it considerably more difficult or even impossible to trace the data. In this manner, extensive and detailed data, as is necessary for the use of artificial intelligence, could be made available while protecting the patients' identity.
So far, however, little research has been done on whether synthetic health insurance data can be used as a high-quality alternative to anonymised original data. In a first step, this project will compare the two strategies together with the Institute for Applied Health Research (InGef) and the Medical Informatics Group of the Berlin Institute of Health (BIH) at Charité - Universitätsmedizin Berlin. Since the HDL is currently still being established, test data from InGef will be used for this purpose. It is important that the data lose as little of the information required for research purposes as possible in the course of the synthetisation process. At the same time, the highest possible level of data protection is aimed at. This is supported by protected virtual environments, so-called "secure processing environments", in which the data will then subsequently be made available. Here, researchers can perform evaluations from their institutes, while the data itself remain at the HDL.
Paving the way for the use of artificial intelligence
The project will then investigate whether and to what extent the anonymised/synthesised health data are suitable for analysis by artificial intelligence ("AI readiness"). It is especially problematic for AI methods when datasets are too small, not representative or too heterogeneous. The Health Data Lab will be facing such challenges when, as of the year 2023, persons insured with the statutory health insurance can release the data of their electronic patient record (ePA) for research purposes. This is made difficult because ePA data is often less structured than the billing data and, if only a few insured persons decide to release their data, AI analyses will become more complicated. Therefore, the HDL and the Fraunhofer Institute for Digital Medicine (MEVIS) are setting up a so-called "sandbox" system, i.e., a virtual room and user-friendly AI toolbox that allows testing specific possibilities in a protected environment. With this option, the HDL is paving the way for applications for research projects requiring AI methods.
These measures also aim at increasing the HDL 's connectedness within the European network. Results obtained can then support the development of European structures for utilising health data within the framework of international initiatives that the HDL is actively involved in (TEHDAS, DARWIN EU).
The research project is funded by the Federal Ministry of Health and is effective until 31 December 2024.
Dr Katharina Schneider
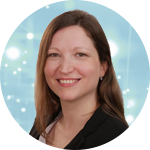
The scientist has been working at the BfArM's Health Data Lab since June 2021. She is involved in the development of the HDL, especially with regard to the project management of national and international further development projects (e.g., KI-FDZ and TEHDAS). Dr Katharina Schneider is a member of the EMA DARWIN EU Advisory Board.
After studying human medicine at the University of Bonn, she was an assistant physician in the field of gynaecology and obstetrics from 2013 to 2014. From 2015 to 2019, she worked as a scientist in the research department of the BfArM with a specialist focus on pharmacogenomics and health services research. From 2019 to 2021, she was an expert at the Federal Office for Social Security (Unit "Data Protection for Supervisory Purposes") where her focus was on data permit activities and the further development of processes regarding transfer of social data for research purposes.